19 Innovation Workbench
Innovation Workbench (IW) is a workspace that provides read-only access to application data objects and clean data by using Oracle APEX. This extension is a workspace for advanced analytics users to add new implementations by using Oracle Advanced Analytic (ODM) algorithms that are implemented as SQL/PLSQL functions. This chapter provides features, examples, and implementation details that are available in Innovation Workbench.
The key features available in Innovation Workbench are:
-
Schema as a Service, in which a user can use AIF cleansed, read-only data, upload and combine data to gain insights, and upload retail application data to further analyze and mine data.
-
Advanced Analytics, allows a user to use Oracle Machine Learning and Advanced Analytic algorithm that are implemented as SQL/PLSQL functions.
-
Visualize Data, enables a user to explore data, develop and visualize data using charts, and review reports using the browser.
-
RESTful Web Service, allows a user to declaratively create and edit RESTful service definitions using SQL Query.
-
SQLWorkshop, enables a user to view and manage database objects.
Components
The key components of Science Innovation Workbench are Retailer Workspace Schema, Oracle APEX, and Oracle Advanced Analytics (Oracle Data Mining).
Figure 19-1 Innovation Workbench Key Components
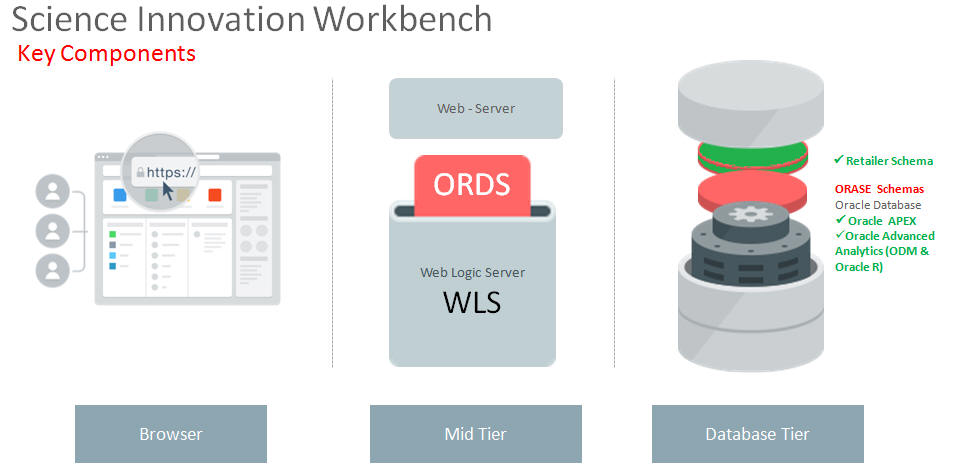
Description of "Figure 19-1 Innovation Workbench Key Components"
Retailer Workspace Schema
Innovation Workbench provides retailer with a logical work area (Retailer Workspace) that is associated with a predefined database schema. The schema(s) store the database objects and provide read access to an existing retailer's application data objects. The retailer workspace schema is an independent schema for a retailer to use and these data objects are owned by retailer.
Oracle APEX
This section describes Oracle APEX, a web-based software development environment.
Workspace
A workspace <RETAILER_WORKSPACE> is a predefined workspace for the retailer where workspace users can create database objects and applications. The workspace has privileges to the allocated <RETAILER_WORKSPACE_SCHEMA> database schema.
Users and Roles
Innovation Workbench has two types of users: application developers and workspace administrators, and they can be created using Oracle APEX.
Innovation Workbench Administrators
The workspace administrator role is already created for the retailer; the administrator can create and edit developer accounts, manage groups, and manage development services.
Innovation Workbench Developer
Workspace administrators can create workbench developers by selecting Manage Users and Groups. Developers can create and modify applications and browse database objects in an allocated workspace and schema. The retailer workspace schema has privileges required by Oracle Data Mining for executing analytic models.
-
Create User. This area displays the Oracle APEX Create User screen that can be used to create a new Developer account and assign a RESTful Service group. Note that this user must also be created in Identity Management with the same username and password.
-
Assign Group RESTful Service.
-
Set Require Change of Password on First Use to No.
SQL Workshop
The SQL Workshop provides tools to view and manage database objects. To create new database objects, click Object Browser and then click Create.
Figure 19-6 SQL Workshop Object Browser: Reading Database Objects
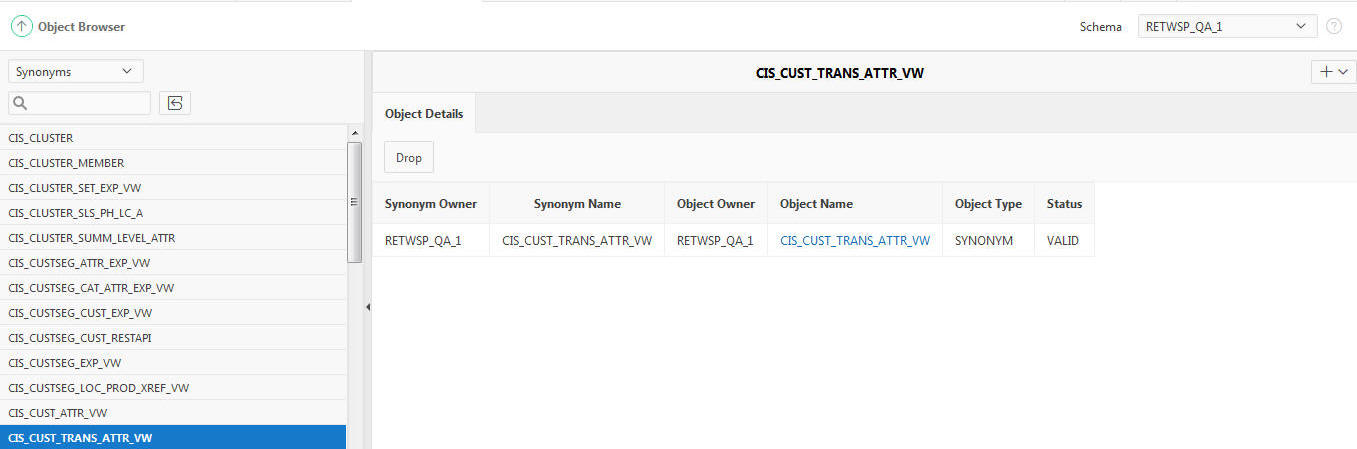
Description of "Figure 19-6 SQL Workshop Object Browser: Reading Database Objects"
Figure 19-8 SQL Workshop SQL Command: Executing Ad Hoc SQLs
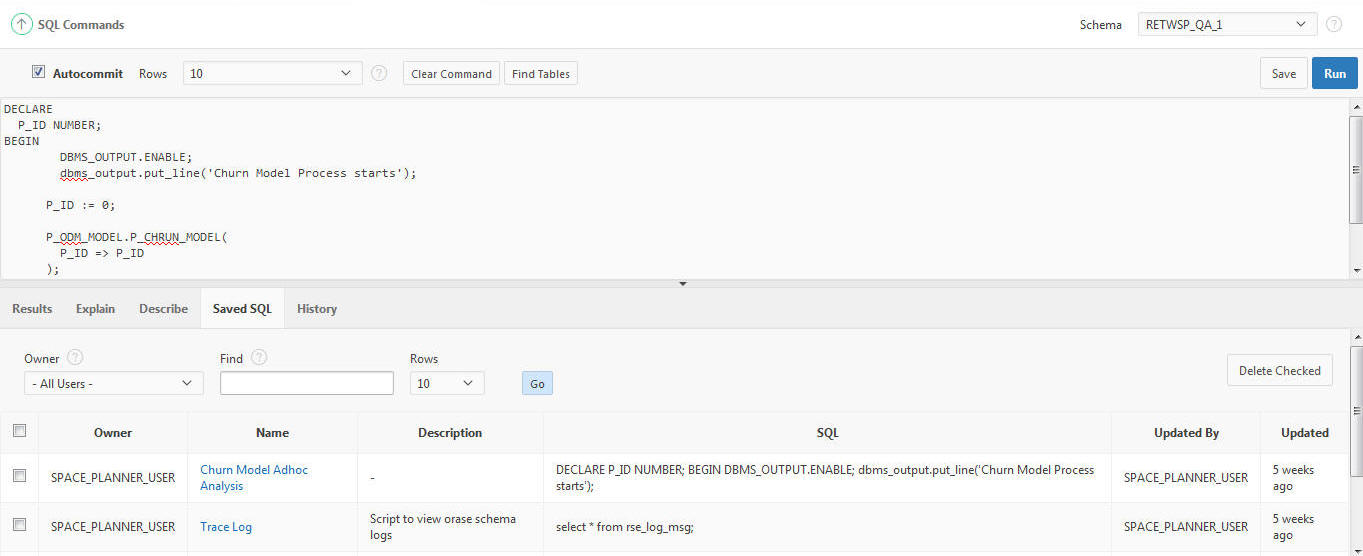
Description of "Figure 19-8 SQL Workshop SQL Command: Executing Ad Hoc SQLs"
Figure 19-9 SQL Workshop SQL Scripts: Uploading, Executing, and Running
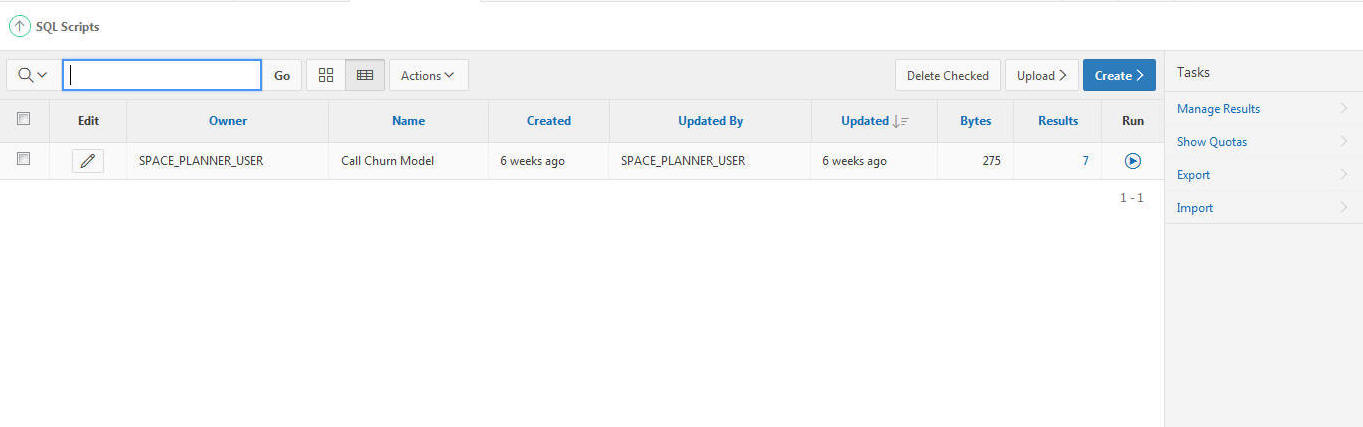
Description of "Figure 19-9 SQL Workshop SQL Scripts: Uploading, Executing, and Running"
Application Builder
Application developers use wizards to declaratively assemble applications organized by page. The session state is transparently managed. Click Create to create an application.
Oracle Advanced Analytics
Oracle Advanced Analytics has, as one of the components in Oracle Database Enterprise Edition, Oracle Data Mining. Innovation Workbench assigns privileges required by Oracle Data Mining for execution.
Oracle Data Mining offers a comprehensive set of in-database algorithms for performing a variety of mining tasks, such as classification, regression, anomaly detection, feature extraction, clustering, and affinity analysis.
Figure 19-11 Machine Learning Algorithms in Database
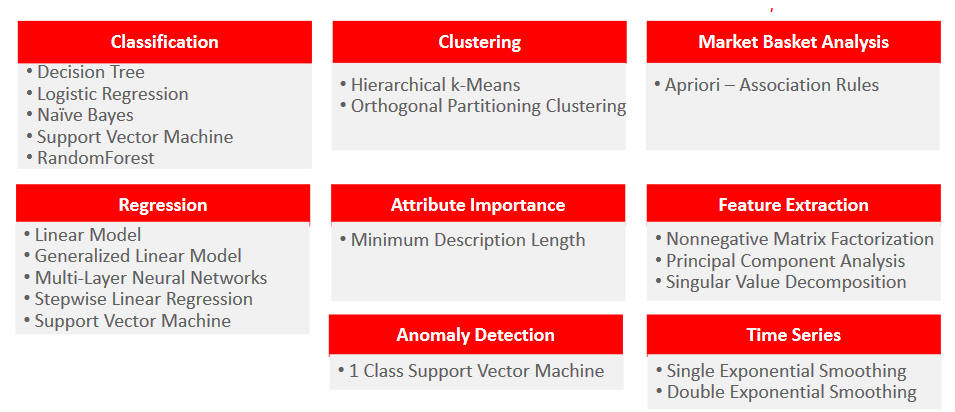
Description of "Figure 19-11 Machine Learning Algorithms in Database"
Oracle Data Mining
Oracle Data Mining can be used to build and deploy predictive and descriptive data mining applications, add intelligent capabilities to existing applications, and generate predictive queries for data exploration.
The Oracle Data Mining developers guide, samples, and tutorials are available at the following websites.
Oracle Data Mining Developer's Guide
http://www.oracle.com/pls/db121/vbook_subject?subject=dma
Data Mining Concepts
https://docs.oracle.com/database/121/DMCON/toc.htm
Oracle Data Mining Sample Programs
http://www.oracle.com/technetwork/database/options/advanced-analytics/odm/odm-samples-194497.html
Samples can be downloaded odm12csampleprograms-2184025.7z
How to Invoke Oracle Data Mining
The following scripts show how to invoke Oracle Data Mining Classification script that creates a classification model using the Decision Tree algorithm.
CREATE OR REPLACE PACKAGE BODY pkg_odm_model AS -- DESCRIPTION - This script creates a classification model using the Decision Tree algorithm. PROCEDURE proc_churn_model( p_id NUMBER) IS BEGIN DECLARE ---------- start drop RETWSP_CUST_CHURN_MODEL not_found EXCEPTION; PRAGMA EXCEPTION_INIT(not_found, -40203); BEGIN dbms_output.put_line('Start Drop RETWSP_CUST_CHURN_MODEL Tables'); DBMS_DATA_MINING.DROP_MODEL('RETWSP_CUST_CHURN_MODEL'); dbms_output.put_line('End Drop RETWSP_CUST_CHURN_MODEL Tables'); EXCEPTION WHEN not_found THEN dbms_output.put_line('RETWSP_CUST_CHURN_MODEL not found'); END; ------------- end drop RETWSP_CUST_CHURN_MODEL -------------------------- -- CREATE A SETTINGS TABLE -- -- The default classification algorithm is Naive Bayes. In order to override -- this, create and populate a settings table to be used as input for -- CREATE_MODEL. -- DECLARE ---------- start drop RETWSP_CUST_CHMDL_SETTINGS not_found EXCEPTION; PRAGMA EXCEPTION_INIT(not_found, -40203); BEGIN dbms_output.put_line('Start Drop RETWSP_CUST_CHMDL_SETTINGS Tables'); EXECUTE IMMEDIATE 'DROP TABLE RETWSP_CUST_CHMDL_SETTINGS'; dbms_output.put_line('End Drop RETWSP_CUST_CHMDL_SETTINGS Tables'); EXCEPTION WHEN not_found THEN dbms_output.put_line('RETWSP_CUST_CHMDL_SETTINGS not found'); END; ------------- end drop RETWSP_CUST_CHMDL_SETTINGS DECLARE ---------- start drop RETWSP_CUST_CHMDL_COST not_found EXCEPTION; PRAGMA EXCEPTION_INIT(not_found, -40203); BEGIN dbms_output.put_line('Start Drop RETWSP_CUST_CHMDL_COST Tables'); EXECUTE IMMEDIATE 'DROP TABLE RETWSP_CUST_CHMDL_COST'; dbms_output.put_line('End Drop RETWSP_CUST_CHMDL_COST Tables'); EXCEPTION WHEN not_found THEN dbms_output.put_line('RETWSP_CUST_CHMDL_COST not found'); END; ------------- end drop RETWSP_CUST_CHMDL_COST DECLARE ---------- start create table RETWSP_CUST_CHMDL_SETTINGS already_exists EXCEPTION; PRAGMA EXCEPTION_INIT(already_exists, -00955); BEGIN dbms_output.put_line('Start Create RETWSP_CUST_CHMDL_SETTINGS Tables'); EXECUTE IMMEDIATE 'CREATE TABLE RETWSP_CUST_CHMDL_SETTINGS ( setting_name VARCHAR2(30), setting_value VARCHAR2(4000))'; dbms_output.put_line('End Create RETWSP_CUST_CHMDL_SETTINGS Tables'); EXCEPTION WHEN already_exists THEN dbms_output.put_line('Exception not found'); END; ------------- end create table RETWSP_CUST_CHMDL_SETTINGS DECLARE ---------- Create RETWSP_CUST_CHMDL_COST Tables begins already_exists EXCEPTION; PRAGMA EXCEPTION_INIT(already_exists, -00955); BEGIN dbms_output.put_line('Start Create RETWSP_CUST_CHMDL_COST Tables'); EXECUTE IMMEDIATE 'CREATE TABLE RETWSP_CUST_CHMDL_COST ( actual_target_value NUMBER, predicted_target_value NUMBER, cost NUMBER)'; dbms_output.put_line('End Create RETWSP_CUST_CHMDL_COST Tables'); EXCEPTION WHEN already_exists THEN dbms_output.put_line('RETWSP_CUST_CHMDL_COST not found'); END; ------------- Create RETWSP_CUST_CHMDL_COST Tables ends -- CREATE AND POPULATE A COST MATRIX TABLE -- -- A cost matrix is used to influence the weighting of misclassification -- during model creation (and scoring). -- See Oracle Data Mining Concepts Guide for more details. -- dbms_output.put_line('Start Insert records into RETWSP_CUST_CHMDL_COST'); DECLARE ---------- sub-block begins already_exists EXCEPTION; PRAGMA EXCEPTION_INIT(already_exists, -00955); BEGIN EXECUTE IMMEDIATE 'INSERT INTO RETWSP_CUST_CHMDL_COST VALUES (0,0,0)'; EXECUTE IMMEDIATE 'INSERT INTO RETWSP_CUST_CHMDL_COST VALUES (0,1,1)'; EXECUTE IMMEDIATE 'INSERT INTO RETWSP_CUST_CHMDL_COST VALUES (0,2,2)'; EXECUTE IMMEDIATE 'INSERT INTO RETWSP_CUST_CHMDL_COST VALUES (0,3,3)'; EXECUTE IMMEDIATE 'INSERT INTO RETWSP_CUST_CHMDL_COST VALUES (1,0,1)'; EXECUTE IMMEDIATE 'INSERT INTO RETWSP_CUST_CHMDL_COST VALUES (1,1,0)'; EXECUTE IMMEDIATE 'INSERT INTO RETWSP_CUST_CHMDL_COST VALUES (1,2,2)'; EXECUTE IMMEDIATE 'INSERT INTO RETWSP_CUST_CHMDL_COST VALUES (1,3,3)'; EXECUTE IMMEDIATE 'INSERT INTO RETWSP_CUST_CHMDL_COST VALUES (2,0,3)'; EXECUTE IMMEDIATE 'INSERT INTO RETWSP_CUST_CHMDL_COST VALUES (2,1,2)'; EXECUTE IMMEDIATE 'INSERT INTO RETWSP_CUST_CHMDL_COST VALUES (2,2,0)'; EXECUTE IMMEDIATE 'INSERT INTO RETWSP_CUST_CHMDL_COST VALUES (2,3,1)'; EXECUTE IMMEDIATE 'INSERT INTO RETWSP_CUST_CHMDL_COST VALUES (3,0,3)'; EXECUTE IMMEDIATE 'INSERT INTO RETWSP_CUST_CHMDL_COST VALUES (3,1,2)'; EXECUTE IMMEDIATE 'INSERT INTO RETWSP_CUST_CHMDL_COST VALUES (3,2,1)'; EXECUTE IMMEDIATE 'INSERT INTO RETWSP_CUST_CHMDL_COST VALUES (3,3,0)'; dbms_output.put_line('End Insert Records'); EXCEPTION WHEN already_exists THEN dbms_output.put_line('RETWSP_CUST_CHMDL_COST not found'); END; ------------- sub-block ends dbms_output.put_line('End Insert records into RETWSP_CUST_CHMDL_COST'); -- Populate settings table DECLARE ---------- sub-block begins already_exists EXCEPTION; PRAGMA EXCEPTION_INIT(already_exists, -00955); v_stmt VARCHAR2(4000); v_algo_name VARCHAR2(100); v_algo_decision_tree VARCHAR2(100); BEGIN dbms_output.put_line('Start Populate settings table' || dbms_data_mining.algo_name); dbms_output.put_line('Start Populate settings table' || dbms_data_mining.algo_decision_tree); v_algo_name := dbms_data_mining.algo_name; v_algo_decision_tree := dbms_data_mining.algo_decision_tree; v_stmt := 'INSERT INTO RETWSP_CUST_CHMDL_SETTINGS (setting_name, setting_value) VALUES (''' || v_algo_name || ''',''' || v_algo_decision_tree || ''')'; dbms_output.put_line('Start Populate settings table v_stmt --' || v_stmt); EXECUTE IMMEDIATE v_stmt; EXCEPTION WHEN already_exists THEN dbms_output.put_line('Exception not found'); END; ------------- sub-block ends DECLARE ---------- sub-block begins already_exists EXCEPTION; PRAGMA EXCEPTION_INIT(already_exists, -00955); v_table_name VARCHAR2(100); v_matrix_cost VARCHAR2(100); BEGIN v_table_name := dbms_data_mining.clas_cost_table_name; v_matrix_cost := 'RETWSP_CUST_CHMDL_COST'; EXECUTE IMMEDIATE 'INSERT INTO RETWSP_CUST_CHMDL_SETTINGS (setting_name, setting_value) VALUES' || '(''' || v_table_name || ''',''' || v_matrix_cost || ''')'; dbms_output.put_line('End Populate settings table'); EXCEPTION WHEN already_exists THEN dbms_output.put_line('Exception not found'); END; ------------- sub-block ends --------------------- -- CREATE A NEW MODEL -- -- Build a DT model dbms_output.put_line('Start Create Churn Model'); DBMS_DATA_MINING.CREATE_MODEL( model_name => 'RETWSP_CUST_CHURN_MODEL', mining_function => dbms_data_mining.classification, data_table_name => 'cis_cust_attr_vw', case_id_column_name => 'CUSTOMER_ID', target_column_name => 'CHURN_SCORE', settings_table_name => 'RETWSP_CUST_CHMDL_SETTINGS'); dbms_output.put_line('End Create Churn Model'); -------------------------- -- DISPLAY MODEL SIGNATURE -- column attribute_name format a40 column attribute_type format a20 SELECT attribute_name, attribute_type FROM user_mining_model_attributes WHERE model_name = 'RETWSP_CUST_CHURN_MODEL' ORDER BY attribute_name; END p_chrun_model; END pkg_odm_model;
Test ODM Model
DECLARE RUN_ID NUMBER; BEGIN DBMS_OUTPUT.ENABLE; dbms_output.put_line('Churn Model Process starts'); RUN_ID := 1001; pkg_odm_model.proc_churn_model( RUN_ID => RUN_ID ); dbms_output.put_line('Churn Model Process ends'); END;
Notebooks
Data scientists can use the Innovation Workbench Notebook to create notebooks, which are collections of documentation, snippets of code, and visualizations. These notebooks are bundled with key python modules for machine learning, data mining, natural language processing, network analysis, and optimization solvers.
Here is a list of some of the python packages that are bundled with AIF. These packages provide features that span data mining processes of from data exploration to data visualization.
-
Ensemble Machine Learning Algorithms with scikit-learn
-
Data exploration and analysis using Pandas; NumPy; SciPy
-
Data visualization using Matplotlib; Seaborn
-
Data storage using cx_Oracle
-
Graph algorithms using Networkx
-
Optimization using Gurobi Solver
Data Studio graph analytics includes numerous built-in graph algorithms. Some of the classes of algorithms that it provides include:
-
Community Detection
-
Path Finding
-
Ranking
-
Recommendation
-
Pattern Matching
-
Influencer Identification
Invoking Python Code
Connecting to a database using cx_Oracle
Database connection string should be fetched from environment variable. Refer to code in Figure 19-13 in red.
Figure 19-13 Connecting to a Database Using cx_Oracle
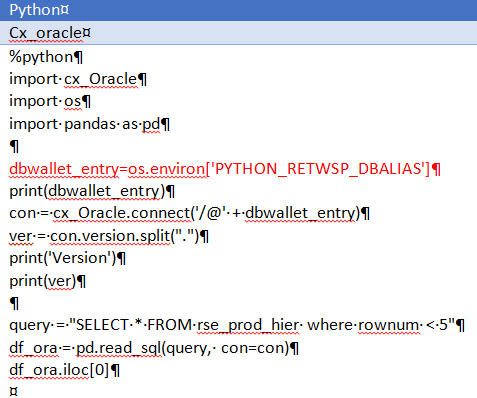
Description of "Figure 19-13 Connecting to a Database Using cx_Oracle"
Executing Gurobi code
Create a Gurobi environment by calling RseGurobiEnv.getGurobiEnv(). Refer to the code in red in Figure 19-14
Invoking Oracle SQL/PL/SQL procedures
Figure 19-15 Invoking Oracle SQL/PL/SQL Procedures

Description of "Figure 19-15 Invoking Oracle SQL/PL/SQL Procedures"
Executing SQL
Executing PL/SQL
Invoking Parallel Graph Analytics
Figure 19-18 Invoking Parallel Graph Analytics

Description of "Figure 19-18 Invoking Parallel Graph Analytics"
Creating a graph from scratch
Scheduling Innovation Workbench Python Notebook
This section describes the scheduling process.
IDCS Setup
In order to use the Datastudio Notebook REST API, the Oracle Identity Cloud Service (IDCS) application is necessary. The IDCS application must be created with following grant_type as (password) and client_credentials.
Once the IDCS application is created, a new clientId:clientSecret will be generated. Note these credentials in order to perform a REST API request. A user must also be created in order to set up the Datastudio User Session.
Scheduling Jobs
Notebooks can be scheduled for automatic execution. To implement this, make a POST request to the using REST API call with the following payloads.
Execute once, immediately.
{ "cronSchedule": "", "timeEnd": "", }
Execute at a regular interval, and stop at a given date.
{ "cronSchedule": "0/1440 * * * * *", "timeEnd": "2021-02-24T21:10:34.400Z", "id": "dsYVGG9Mvw" }
Execute at a regular interval, indefinitely.
{ "cronSchedule": "0/ 1440 * * * * *", " timeEnd": "", "id": "dsYVGG9Mvw" }
REST API Documentation
The REST API documentation details for request/response are located here:http://datastudio.oraclecorp.com/docs/apidoc/swagger-ui.html#/
Example
Here is a REST API curl call example showing how to schedule a notebook.
Table 19-1 Example for Scheduling Notebooks
Seq. # | REST API Purpose | REST API Curl Request | REST API Curl Response |
---|---|---|---|
1 |
Fetch Authentication TokenPOST request |
curl --location --request POST 'https://<IDCS_HOST>/oauth2/v1/token' \--header 'Authorization: Basic <Base64 ClientID:ClientSecret>' \--header 'Content-Type: application/x-www-form-urlencoded' \--data-urlencode 'grant_type=password' \--data-urlencode 'scope=urn:opc:idm:__myscopes__' \--data-urlencode 'username=<username>' \--data-urlencode 'password=<password>' |
Response will have authentication token. To make subsequent request: { "access_token": "<Access Token>", "token_type": "Bearer", "expires_in": 3600} |
2 |
Setup SessionGET request |
curl --location --request GET '<APP_HOST>/datastudio/v2/sessions/user' \--header 'Authorization: Bearer <Token>' |
{ "username": "<user_name>", "permissions": [ "graph_create", "export_all", "view_permissions_tab", "import_notebook", "view_dashboard_tab", "view_credentials_tab", "create_notebook", "create_credential", "view_interpreter_tab", "delete_all" ], "authToken": "<Token>"} |
Note: The first two steps above are mandatory. Execute any of the following steps. |
|||
3 |
Schedule NotebookPOST request |
curl --location --request POST 'https://<APP_HOST>/datastudio/v2/notebooks/schedule' \--header 'Authorization: Bearer <Token>' \--header 'Content-Type: application/json' \--data-raw '{ "cronSchedule": "", "timeEnd": "", "id": "dsYVGG9Mvw"}' |
{ "id": "dsja4YWg", "status": "SUBMITTED", "startTime": null, "endTime": null, "error": null, "tasks": []} |
4 |
Schedule Notebook Paragraphs to execute immediatelyPOST request |
curl --location --request POST 'https://<APP_HOST>/datastudio/v2/notebooks/schedule' \--header 'Authorization: Bearer <Token>' \--header 'Content-Type: application/json' \--data-raw '{ "cronSchedule": "", "paragraphs": [ { "id": "dsB0zM58" } ], "timeEnd": "", "id": "dsYVGG9Mvw"}' |
{ "id": "dsVBqlO3", "status": "SUBMITTED", "startTime": null, "endTime": null, "error": null, "tasks": []} |
5 |
Schedule Notebook to execute immediately.Execute a notebook every five minutes.POST request |
curl --location --request POST 'https://<APP_HOST>/datastudio/v2/notebooks/schedule' \--header 'Authorization: Bearer <Token>' \--header 'Content-Type: application/json' \--data-raw '{ "cronSchedule": "*/1440 * * * * *", "endDate": "2020-12-17T14:10:34.400Z", "id": "dsYVGG9Mvw"}' |
{ "id": "dsja4YWg", "status": "SUBMITTED", "startTime": null, "endTime": null, "error": null, "tasks": []} |
6 |
Schedule Notebook Paragraphs.Execute a notebook/paragraph every five minutes.POST request |
curl --location --request POST 'https://<APP_HOST>/datastudio/v2/notebooks/schedule' \--header 'Authorization: Bearer <Token>' \--header 'Content-Type: application/json' \--data-raw '{ "cronSchedule": "*/1440 * * * * *", "paragraphs": [ { "id": "dsB0zM58" } ], "timeEnd": "2020-12-17T14:10:34.400Z", "id": "dsYVGG9Mvw"}' |
{ "id": "dsVBqlO3", "status": "SUBMITTED", "startTime": null, "endTime": null, "error": null, "tasks": []} |
7 |
Schedule Notebook Paragraphs.Execute a notebook/paragraph adhoc with parameters. |
curl --location --request POST 'https://<hostname>/datastudio/v2/notebooks/schedule' \--header 'Authorization: Bearer <Token>' \--header 'Content-Type: application/json' \--data-raw '{ "cronSchedule": "", "paragraphs": [ { "id": "dsa7kZYv", "message": "string", "params": { "FirstName": "Sandhya", "LastName": "Lonial" } } ], "timeEnd": "2020-02-24T21:10:34.400Z", "id": "dsYVGG9Mvw"}' |
{ "id": "dsOB0yra", "status": "SUBMITTED", "startTime": null, "endTime": null, "error": null, "tasks": []} |
8 |
Schedule Notebook Paragraphs.Execute a multiple paragraphs. |
{ "cronSchedule": "", "paragraphs": [ { "id": "dsB0zM58" }, { "id": "dsa7kZYv", "message": "string", "params": { "FirstName": "Sandhya", "LastName": "Lonial" } } ], "timeEnd": "2020-02-24T21:10:34.400Z", "id": "dsYVGG9Mvw"} |
{ "id": "dsOB0yra", "status": "SUBMITTED", "startTime": null, "endTime": null, "error": null, "tasks": []} |
Notebook and Paragraph IDs
To obtain notebook and paragraph IDs, select a paragraph, using the Setting Context menu. Click Open as Iframe. A new browser tab opens. Copy the url in the address bar in browser and borrow IDs from the URL notebookId=dsYVGG9Mvw&pid=dsB0zM58
https://<host>/datastudio/?root=iParagraph¬ebookId=dsYVGG9Mvw&pid=dsB0zM58&readOnly=false&showCode=true
Considerations
Consider the following:
-
Make sure there are no empty paragraphs as this results in an 500 error response.
-
To test, create a table and view records that are inserted in the table.
%oracle insert into iw_test (first_name, last_name ) values (John, 'Doe')
%python print('Hello World')
Verify using the following:
CREATE TABLE iw_test ( person_id NUMBER GENERATED BY DEFAULT AS IDENTITY, first_name VARCHAR2(50) NOT NULL, last_name VARCHAR2(50) NOT NULL, PRIMARY KEY(person_id) ); select count(*) from iw_test;
REST API
Here is a REST API curl call example showing how to schedule a notebook.
Table 19-2 Example
REST API purpose | REST API curl request | REST API curl response |
---|---|---|
Fetch Authentication Token |
curl --location --request POST 'http://bur00afq.us.oracle.com:26000/datastudio/v2/sessions/login' \--header 'Authorization: Basic b3Jhc2UxOnBhc3N3b3JkMQ==' \--header 'Content-Type: application/json' \--header 'Cookie: JSESSIONID=cmWRE8eaMH1e7ClA72Qx8QVxX3SKVMuEi1S_ErDgySh2qInJRbdZ!1637160649' \--data-raw '{"credentials":"password1","principal":"orase1"}' |
Response will have authentication token. To make subsequent request:authToken":"de6460f5-e36c-4592-b2c3-6ef18268e6c5" |
Schedule Notebook |
curl -X POST -H 'Content-Type: application/json' -H 'x-auth-token: de6460f5-e36c-4592-b2c3-6ef18268e6c5' -i 'https:// <host>:<port>/datastudio/v2/notebooks/schedule' --data '{ "cronSchedule": "0/5 * * * * *", "endDate": "2020-02-24T21:10:34.400Z", "id": "dsZgvK3G"} |
{"id":"dsbBVLgL","status":"SUBMITTED","startTime":null,"endTime":null,"error":null,"tasks":[]} |
Restful Service
RESTful Services allow for the declarative specification of RESTful access to the database. They are created by configuring a set of Uniform Resource Identifiers (URIs) to a SQL query or anonymous PL/SQL block. The set of URIs is identified by a URI template.
To create a RESTful service:
-
Select SQL Workshop RESTful Services.
-
On selecting RESTful Services, you see the option to create a RESTful Service.
-
A RESTful Service Module is a grouping of common templates, known as Resource Templates, under a common URI prefix. This prefix is prepended to all templates.
-
A URI template is a simple syntax for describing URIs. You populate the required fields as shown in Figure 19-24 and make sure to set the required privileges as Rest Privileges.
-
A Resource Handler is a query or an anonymous PL/SQL block responsible for handling a particular HTTP method. Multiple handlers can be defined for a Resource Template; however, only one handler per HTTP method is permitted. You can select method, source type, format, and SQL Query to read data from the schema.
-
To test the rest API, click Test Button (see Test at the bottom right corner.)
-
The page refreshes and displays data in JSON format.
Integration
IW provides a customer with a way to integrate with other applications. A framework is provided that enables it to receive and read-in files from customers so that the data contained can be loaded into IW tables. They are thus able to use data from their other systems within IW itself.
Consequently, data within IW can also be exported to flat files so that their other applications can use the AIF-cleansed data that is available via IW.
More details on the framework for these capabilities are contained in the following Custom Data Loads and Custom Data Exports sections.
Custom Data Loads
AIF provides functionality to load data files from a customer site sent via Secure FTP, from which all other daily interface files are sent. A zip file, which can contain any number of files, can be provided, along with the specifications about the files to be loaded. (These files are referred to as context files here.) Once the data has been sent, a process is invoked from the Process Orchestration and Monitoring (POM) application to initiate loading the data into the appropriate tables inside the Innovation Workbench. Once the data load is complete, a zip will be uploaded to Object Storage to provide the data load logs, which will indicate whether or not there were any rejected records.
Required Files
The following are the prerequisites for performing a data load:
-
The table must exist inside the Innovation Workbench.
-
A zip file named ORASE_IW_DATA.zip must be created. It must contain the data to be loaded and the context files that describe details about the data load.
-
The ORASE_IW_DATA.zip file must be uploaded to the Object Storage using the File Transfer Service (FTS).
Zip File Contents
The ZIP file must contain a pair of files for each table to be loaded, a .dat file and a .ctx file.The data file can have any name, with the .dat extension. The context file must match the name of the data file, but with the .ctx extension. If a data file is provided without a .ctx file, then the data file will be ignored.
For example, to load a table called STG_WKLY_SLS, you require a file called STG_WKLY_SLS.dat to hold the data to be loaded and a file called STG_WKLY_SLS.dat.ctx to describe the details regarding the load.
No support exists for directory names inside the zip file, so if they are provided they will be ignored. For example, you cannot send directory1/STG_WKLY_SLS.dat and directory2/STG_WKLY_SLS.dat because they will end up overriding each other when the zip is extracted.
Although you can provide multiple files to be loaded in a single zip, none of them can be for the same table as another load. The load truncates the data in the table to be loaded, so if the same table is targeted by multiple files, then only the latest file will be loaded. Because this data is truncated prior to each load, you should follow a pattern in which these tables are the staging tables to be used to then populate data in other tables. In this way, the data is verified, which allow for the merging of data, via updates or inserts. This is not possible if you loaded directly to your final table.
Context File Details
The .ctx file provide options for including details that describe the data load. These details are similar to the options used by SQL*Loader, so refer to documentation about the SQL*Loader for any additional details about concepts noted here.
Here is an example of a context file.
#TABLE#SLS_WKLY_SLS# #DELIMITER#|# #COLUMN#WEEK_DATE#DATE(10) "YYYY-MM-DD"# #COLUMN#PRODUCT_KEY# #COLUMN#LOCATION_KEY_FILLER#BOUNDFILLER# #COLUMN#SLS_AMT# #COLUMN#LOCATION_ID#"some_db_package.lookup_location_id(:LOCATION_KEY_FILLER)"# #COLUMN#LOAD_DATE#"sysdate"#
In the above example contextual file:
-
The #TABLE## line is required in order to specify the name of the table to be loaded.
-
The #DELIMITER## line is optional, and if not provided, then a | will be considered the default value.
-
The table to be loaded will be loaded via a Direct Path load (for efficiency sake). It will truncate the table prior to loading the table. This means that you cannot provide multiple files in a single transmission for the same table.
-
The columns can be wrapped in " " if necessary.
-
The data in the file must be provided as CHARACTERSET AL32UTF8.
-
For the column specifications, the columns must be listed in the order in which they appear in the file.The format is #COLUMN#THE_COLUMN_NAME#Any special load instructions#
-
Note that for Date columns, you must provide a proper date specification with the format that you are providing the data in.The example above for WEEK_DATE illustrates how to specify the date in a YYYY-MM-DD format.
-
For numeric columns, you do not normally require any additional load instructions.
-
If you are providing data that must be used as a BOUND FILLER during the load of another column, then you must specify BOUNDFILLER, just like you would in SQL*Loader.
-
The LOCATION_ID example above illustrates how you can refer to a FILLER column, which is then used as a parameter to a function, which then returns the actual value to load to the column.
-
If you want an value such as the system date/time to be loaded, then you can specify "sysdate" as shown above for LOAD_DATE column.
-
When you are populating columns that are loaded via a special expression (such as LOCATION_ID and LOAD_DATE above), be sure to provide them last in the context file.
-
When loading data character data, it maybe be necessary to specify the column like this: CHAR(50). This is commonly required by SQL*Loader when advanced character sets are used.
-
The examples shown above for LOCATION_KEY_FILLER and LOCATION_ID are not usual/simple use cases, but are supported. For a better understanding of how that works, refer to the documentation for SQL*Loader.
Data Load Feedback
When the data load is complete, a zip file named ORASE_IW_DATA_extract.zip will be uploaded to Object Storage and it will contain the log and bad files for the data load.
Invoking the Data Load
In order to invoke the load process, you must use the POM application to invoke the following adhoc process:
RSE_LOAD_IW_FILES_ADHOC.
This controls the execution of all these steps. Once the load has been completed, you should be able to use the data as necessary inside the Innovation Workbench. See "Process Orchestration and Monitoring" for additional details.
Custom Data Exports
You can export data that has been created within the Innovation Workbench workspace. You first configure the table to be exported. A process is then executed that exports the table data to a text file. The data is then gathered into a zip file, and the zip file is moved to the Object Storage for retrieval using File Transfer Service (FTS).
Required Configuration
Using the Data Management menu option (described in Oracle Retail Science Cloud Services User Guide), you can manage the tables to be exported, using the formatting shown in Table 19-3. Once in the screen, select the table RSE_CUSTOM_EXP_CFG. Then, add rows and make adjustments to existing rows as required in order to define the tables to be exported.
Table 19-3 Export Table Formatting
Column Name | Data Type | Example | Comments |
---|---|---|---|
TABLE_NAME |
Character(30) |
IW_RESULTS_TABLE |
The name of the table to be exported. |
FILE_NAME |
Character(80) |
iw_results_table.csv |
The name of the file to be created, excluding any directory names. |
DESCR |
Character(255) |
Results table of XYZ Calculation |
Any description to describe the table. |
COL_DELIMITER |
Character(1) |
, (comma) |
The character to use as a delimiter between columns. |
COL_HEADING_FLG |
Character(1) |
Y |
A Y/N value to indicate if the export should include a heading row (Y) that contains the names of the columns, or not (N). |
DATE_FORMAT |
Character(30) |
yyyy-MM-dd HH:mm:ss |
The format to use for any date columns. This format must be a valid format for Java date handling. |
ENABLE_FLG |
Character(1) |
Y |
A flag to indicate if this table should be exported (Y) or not (N) This flag can be used to temporarily disable an export, without the need to remove it completely. |
Invoking the Export
In order to begin the export process, you must use the POM application to invoke the following AdHoc process:
RSE_IW_EXPORT_FILES_ADHOC / job: RSE_IW_EXPORT_FILES_ADHOC_JOB.
This process controls the execution of the steps to export the data. Once the export has been completed, a zip file named ORASE_IW_EXPORT_extract.zip is created. All files will be named according to the names specified in the RSE_CUSTOM_EXP_CFG table. See "Process Orchestration and Monitoring" for additional details on how to execute a Standalone/AdHoc Sample Extensibility Use Caseprocess.
Sample Extensibility Use Case
Clients can use IW's framework to address use-cases unique to their business requirements.
Here is one possible example of IW's extensibility.
A customer wants to expose AIF-cleansed sales transaction data from AI Foundation to a third-party system, using an integration software for connecting applications, data, and devices. This enables their other applications to access the exact calculations/AIF-cleansed data that they require.
IW can be extended as outlined below in order to address this use-case:
-
Load sales data into AI Foundation using the existing nightly sales data load routine.
-
Create a custom PL/SQL package to transform the data as required and load the transformed data into custom tables in IW. For example, sales can be aggregated per customer/transaction. This aggregated data is loaded into the custom IW table, with specific custom keys required to identify the records in external systems.
This custom PL/SQL can include status tracking using auxiliary tables to capture the status of the executed routine, as well as log pertinent information that can enable re-execution if necessary.
-
Create ReST APIs in APEX that reference the custom IW tables, to expose the sales transaction data.
-
Create and schedule load jobs in APEX that invoke the custom PL/SQL package to populate the custom tables. These daily programs must be scheduled using DBMS_SCHEDULER jobs that are aligned with the expected end-time of the daily Retail Insights batch.
-
Real-time ReST API calls can then be made from the integration software and then into the third-party system that will use the data.
Troubleshooting
Science Innovation Workbench can also be used for monitoring application logs to troubleshoot issues in an application process due to an error in a batch or business process.
-
Click Innovation Workbench.
-
Select Retailer Workspace.
-
Select SQL Workshop.
-
Select SQL Command.
-
Enter SQL select * from rse_log_msg order by msg_ts desc;
-
Select run and view the log message in the bottom panel.
-
You can describe objects using describe rse_log_msg;
-
DBMS Scheduler
Innovation Workbench can be used for data mining tasks that are long running and have to rely on database scheduling functions and procedures that can be called from any PL/SQL program.
Any jobs created in the Retailer Workspace using Innovation Workbench must be created under Job Class RETAILER_WORKSPACE_JOBS. Jobs created in a default job class or any other job class other than RETAILER_WORKSPACE_JOBS can be disabled by an Oracle Administrator while managing resources.
BEGIN DBMS_SCHEDULER.CREATE_JOB ( job_name => 'retwsp_churn_model', job_type => 'STORED_PROCEDURE', job_action => 'retwsp.pkg_customer_analytics.proc_churn_model', start_date => '01-JAN-17 07.00.00 PM US/Pacific', repeat_interval => 'FREQ=YEARLY; BYDATE=0331,0630,0930,1231; ', end_date => '31-DEC-17 07.00.00 PM US/Pacific', job_class => 'RETAILER_WORKSPACE_JOBS', comments => 'Retailer workspace churn model job'); END; /
Schema Objects
Database objects owned by the various schemas in AI Foundation are available for the advanced analyst to use. Here are some examples:
Table 19-4 Schema Objects
Table Name | Description |
---|---|
rse_cal_hier |
This table is used to hold all calendar hierarchies. Examples are the normal calendar hierarchy, and can also contain an alternate hierarchy for the fiscal calendar. |
rse_prod_hier |
This table is used to hold all product hierarchies. Examples are the normal product hierarchy, and can also contain an alternate category hierarchy. |
rse_loc_hier |
This table is used to hold all location hierarchies. Examples are the normal organizational hierarchy, and can also contain an alternate hierarchy for trade areas. |
rse_prod_loc_status |
Status of the item at this location for this time frame. A-Active; I-Inactive; C-Discontinued; D-Deleted |
rse_ret_lc_wk_a |
This table contains aggregate sales data for the dimensions of a location and a week. |
rse_sls_lc_wk_a |
This table contains aggregate sales data for the dimensions of a location and a week. |
rse_sls_pr_lc_cs_wk |
This table contains aggregate sales data for a Product, Location, Customer Segment and Week. The SLS_PR columns represent the metrics for that week that were on promotion, while the other metrics represent the sales metrics while the item was not on promotion. |
rse_sls_pr_wk_a |
This table contains aggregate sales data for the dimensions of a product and a week. |
rse_sls_ph_lc_wk_a |
This table contains aggregate sales transaction data for different product hierarchy/levels, at the store location/week dimension. |
rse_sls_pr_lc_wk |
This table contains aggregate sales data for a Product, Location, and Week. The SLS_PR columns represent the metrics for that week that were on promotion, while the other metrics represent the sales metrics while the item was not on promotion. |
rse_sls_txn |
This table contains sales transaction data. |
rse_prod_attr |
This is the table that holds product attributes. |
rse_prod_attr_grp |
This is the table used to load the associations of CM Groups to product attributes. |
rse_prod_attr_grp_value |
This is the table used to load the associations of CM Groups to product attributes and its values |
rse_prod_attr_grp_value_map |
This is the table used to load the associations of CM Groups to product attributes, group values and actual product attribute values |
rse_like_loc |
This is the table used to load the like stores for CMGroup or Category. |
rse_hier_level |
This table defines the various levels for all the hierarchies. |
rse_hier_type |
This table defines the available hierarchies for use within the RSE applications. |
rse_fake_cust |
Table for specifying customers who are considered as fake customers. A fake customer is a customer who purchases too many transactions to be considered a single customer. Examples are generic store cards. |
rse_loc_src_xref |
This table contains integration ID information that enables interaction with other systems, using IDs that other systems can accommodate for the Location Hierarchy. |
rse_prod_src_xref |
This table contains integration ID information that enables interaction with other systems, using IDs that other systems can accommodate for the Product Hierarchy. |
rse_log_msg |
This table contains messages logged while database, batch or business processing. |
w_party_per_d |
This table contains customer data and its attribute. |
cis_cust_attr_vw |
Customer Attributes - This view provides basic customer attributes. |
cis_cust_trans_attr_vw |
Customer Transaction Attributes - This view provides attributes for customer transactions. |
cis_cust_trans_ph_attr_vw |
Customer Transaction Attributes - This view provides product attributes for customer transactions. |
cis_custseg_attr_exp_vw |
This view provides an export of the attributes that define a segment. |
cis_custseg_cat_attr_exp_vw |
This view provides an export of the product attributes that define a segment. |
cis_custseg_cust_exp_vw |
This view provides the members for an exportable set of segments. |
cis_custseg_exp_vw |
This view provides an exportable set of clusters for customer segmentation. |
cis_sls_cust_cal_a |
This table contains aggregate customer sales data for a configurable level of the calendar hierarchy. The table is to be partitioned by Calendar and is also be suitable for sub partitioning by Customer using a Hash Partition strategy, so that subsequent uses can operate within the confines of a given Hash partition. |
cis_sls_cust_ph_cal_a |
This table contains aggregate customer sales data for a configurable level of the calendar hierarchy, for a selected set of product hierarchies. The table is to be partitioned by Calendar and is also be suitable for sub-partitioning by Customer using a Hash Partition strategy, so that subsequent uses can operate within the confines of a given Hash partition. |
cis_sls_ph_a |
This table contains aggregate sales data for all product hierarchy members of a configured hierarchy type and level. This can be used to identify the Top Categories for use by things like Customer Segmentation. |
cis_cluster_set_exp_vw |
This view provides an exportable set of clusters to send to Cat Man. |
cis_store_cluster_exp_vw |
This view provides an exportable set of clusters for stores. |
cis_store_cluster_mem_exp_vw |
This view provides the members with an exportable set of segments. |
cis_store_cluster_prop_exp_vw |
This view provides an exportable set of clusters for stores. |
cis_cluster_sls_ph_lc_a |
This table contains calendar level aggregates for the various clusters. The table is to be partitioned by Calendar. |
cis_cluster_summ_level_attr |
These are metrics generated at cluster/attribute/business object level. There are metrics that are generated at that level such as centroid. |
cis_prod_attr_loc_share |
This table contains aggregate sales data for product attribute values as well as the share that these values are with respect to the total sales for the product hierarchy, calendar, location. The share value is a configurable value, which can either be based on sales units, sales amount or profit amount. |