B Appendix: Forecast Errors in the Forecast Scorecard Dashboard
This appendix provides background and formulas for the error metrics used in the Forecast Scorecard dashboard. The errors are always calculated at the lowest level - typically item/store/week, and then averaged at the intersection of the dashboard tiles. For GA this intersection is subclass/district, but it can be configured at implementation time. The evaluation of the forecast is done over a window starting today and looking back a configurable number of periods. All errors are calculated for the system-generated forecast as well as the user adjusted forecast.
The following are the error metrics:
Mean Absolute Percent Error
The percentage error of a forecast observation is the difference between the actual POS value and the forecast value, divided by the actual POS value. The result of this calculation expresses the forecast error as a percentage of the actual value. The Mean Absolute Percentage Error statistic measures forecast accuracy by taking the average of the sum of the absolute values of the percentage error calculations across all observations. This method is useful when comparing the accuracy of forecasts for different volume products (it normalizes error by volume).
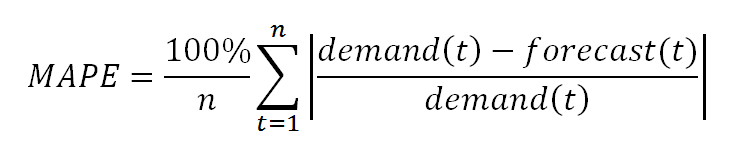
Root Mean Squared Error
This is the square root of the Mean Squared Error. The Root Mean Squared Error is one of the most commonly used measures of forecast accuracy because of its similarity to the basic statistical concept of a standard deviation. It evaluates the magnitude of errors in a forecast on a period-by-period basis, and it is best used to compare alternative forecasting models for a given series.
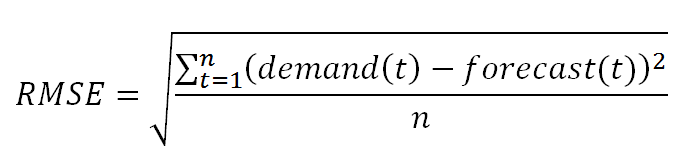
Mean Absolute Error
The absolute error of a forecast observation is the absolute value of the difference between the forecast value and the actual POS value. The Mean Absolute Error statistic is a measure of the average absolute error. This is calculated by summing the absolute errors for all observations and then dividing by the number of observations to obtain the average. Mean Absolute Error gives you a better indication of how the forecast performed period by period because the absolute value function ensures that negative errors in one period are not canceled out by positive errors in another. Mean Absolute Error is most useful for comparing two forecast methods for the same series.
